Much has been said and written about generative AI (Gen AI), ChatGPT, and large language models in the last couple of months. Awe and excitement, as well as fear that these systems will take over human creativity, which is perceived as the fundamental prerogative of humankind. Data specialists criticized the reliability and truthfulness of such learning models, pointing out that these platforms can be used for malicious purposes like fake news and disinformation, trolling, plagiarism, and others.
So, we decided to dive deeper into what generative AI systems are, how they work, their perks, and their limitations. And since there is a great deal of information to chew, it's better to serve it one piece at a time. Thus, this blog post is part of a series of blogs we will publish around this hot topic.
If you are wondering whether bots are stealing human creativity, the answer is neither true nor false. It works like the learning models upon which Gen AI is based: the better you train them, the smarter they get. They contain, in their neural circuitry, a generator and a critic. Not an easy robot life, is it?
What is Generative AI?
Generative AI is a branch of artificial intelligence that focuses on producing new content. It takes input from large datasets or algorithms and applies them to the problem at hand. By doing so, it produces unique results such as images, texts, audio, etc.
Gen AI has the potential to become the next unicorn of AI technology.
This picture was generated with Midjourney, an AI system that creates realistic images and art from a given textual description.
How Does Gen AI Work?
One could think that this definition is enough. We weren’t convinced, so we researched how generative AI systems work. It turns out that if you take a multilayered artificial brain and feed it with great amounts of data, then apply machine learning algorithms to train it, the brain will be able to create novel content in response to random input. Learning from iterations is something AI gets good at - unlike humans, as history proved. This capability is due mainly to the generator component.
Nevertheless, if that were so easy, we wouldn’t spend time decomposing generative AI’s artificial brain, the Generative Adversarial Network. As soon as a certain type of content is generated, the discriminator (or critic) comes to life to evaluate the output against given criteria – remember the machine learning algorithms? – and to determine its quality and accuracy. It can be a “go-go” or a “no-go”; it all depends on the critic’s evaluation… we all have our internal struggles, don’t we?
The process of machine learning happens when both components are trained simultaneously via optimization algorithms that help improve performance over time as both components come to peace to produce more accurate results. The generator learns to create more realistic data samples, while the discriminator learns to distinguish real samples from fake ones created by the generator. As they become increasingly better at generating new content within a given domain, they also become increasingly better at distinguishing between right and wrong.
Let's take, for example, image-to-image translation. GANs can learn to map patterns from an input image to an output image. For example, it can be used to transform an image into a specific artistic style, to age the image of a person, or for many other image transformations. The same goes for industrial design and architecture, where generative AI systems can create new 3D product designs based on existing products. For example, a GAN can be trained to create new furniture designs or propose new architectural styles.
That being said, generative AI has the great potential to create new pieces of content, having many diverse applications – from generating realistic images to creating natural-sounding music. Sequoia predicts that by 2030, generative AI will be able to put together scientific papers and visual design mock-ups, write, design, and code smarter and more efficiently than humans. Businesses can use it to produce content, such as articles, whitepapers, press releases, blogs, or social media posts.
To make its usage as friendly and natural as possible, OpenAI launched ChatGPT, and the creators' bubble shattered again. Would that be the end of creativity as we know it?
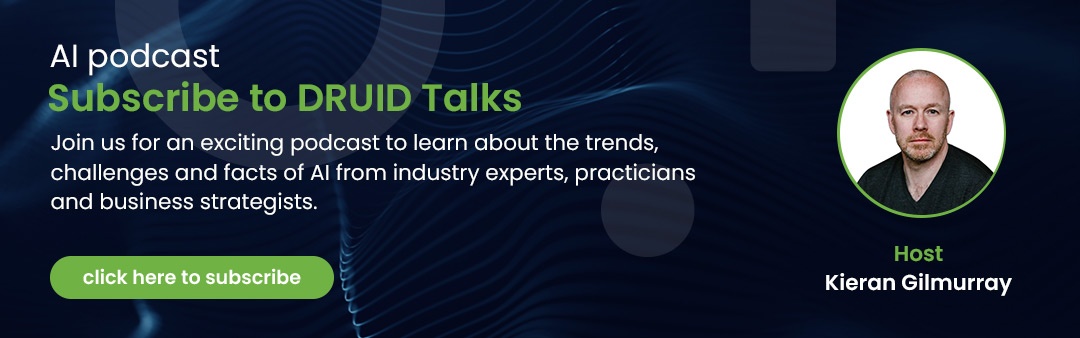
Is ChatGPT the Best Expression of Generative AI so Far?
To understand why ChatGPT is the ultimate piece of technology creating thrills in the generative AI world, let’s dive into what it is and can do.
What is ChatGPT?
The simple definition is that it’s a type of generative AI which uses natural language processing (NLP) and deep learning algorithms to generate responses in the form of text or images. What makes it spectacular is that it generated 1 million users in less than a week, a number that took Netflix, for example, 3.5 years to reach. What gives it flavour is that it comes under the form of a chatbot – so the interaction is natural – that can answer pretty much anything asked and understands the question, the content, and the context. How come?
What is a Large Language Model?
ChatGPT is developed on a large language model (LLM) named GPT-3.5 and developed by OpenAI, which is said to be one of the largest and most advanced language models currently available. Large Language Models (LLMs) are artificial intelligence tools that use natural language understanding (NLU) and natural language generation (NLG) to read, summarize, and translate texts that predict future words in a sentence. The model is constantly improved and optimized for conversational dialogue using Reinforcement Learning with Human Feedback (RLHF).
Why is ChatGPT special?
Although ChatGPT is not connected to the internet, it has been trained on an enormous amount of historical data (pre-2021) – not confirmed. It is believed to be in the range of hundreds of billions of words and presents its findings in an easily consumable way.
If it hasn't dazzled you yet, here is another secret: it's the massive amount of data to which the model was exposed that enabled researchers to train the model to understand the nuances and subtleties of human language and generate responses that are coherent and grammatically correct.
Moreover, the quality of data it was fed with, incorporating a wide range of topics and styles, allows the model to generate relevant responses to a certain context or situation. Not only can it provide accurate and fast responses, but it can also learn from the conversation it has with a user, allowing it to continue learning and improving its prediction accuracy over time. This unique capability allows it to provide better predictive results for longer conversations without re-training. Thus, it's ideal for a wide range of applications, such as conversational AI agents, fictional writing, text-to-speech systems, image generator systems etc.
The chatbot in ChatGPT allows interaction in a seemingly ‘intelligent’ conversational manner, while GPT3 produces output that appears to have ‘understood’ the question, the content and the context. Together this creates an uncanny valley effect of "Is it human or a computer? Or is it a human-like computer?" The interaction is sometimes humorous, sometimes profound and sometimes insightful.
Apparently, it's not shy when enumerating its capabilities, either.
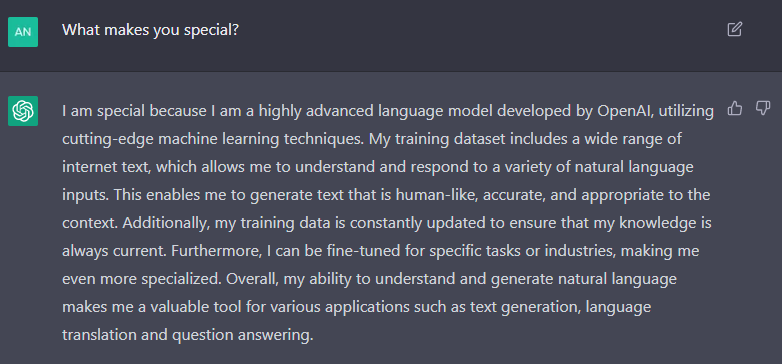
Unfortunately, the content is also sometimes incorrect and is never based on a human-like understanding or intelligence. The problem may be with the terms "understand" and "intelligent." These are terms loaded with implicitly human meaning, so when applied to an algorithm, it can result in severe misunderstandings. The more useful perspective is to view chatbots and large language models (LLM) like GPT as potentially useful tools for accomplishing specific tasks and not as parlour tricks. Success depends on identifying the applications for these technologies that benefit organizations.
Looking into the Future
By 2025, Gartner estimates that generative AI will produce 10% of all data. It will contribute towards 50% of pharmaceutical discovery/development, and by 2027, 30% of manufacturers will use generative AI to enhance their product development effectiveness.
Generative AI is having its moment, and we’ll start to see many more products and services come to market in 2023. Taking advantage of AI-powered language applications and using enhanced language understanding capabilities to build conversational AI agents capable of completing business tasks is happening as we speak.
Generative AI models are incredibly diverse. They can be fed with images, longer text formats, emails, social media content, voice recordings, program code, and structured data. They can output new content, translations, answers to questions, sentiment analysis, summaries, and even videos. These universal content machines have many potential applications in business. For example, they can be used for marketing applications (like Jasper.ai), code generation apps (like Microsoft-owned GitHub Copilot), knowledge management apps, and conversational AI (Google's BERT or Meta's BlenderBot).
Nevertheless, it’s still nascent technology; ChatGPT, in its current form, is best used for creative endeavours like content creation and ideation. It is still not great at things like precise answers to internal content or complex math equations. It doesn't mean that over the next years, generative AI won't make an impact in traditional industries such as manufacturing, healthcare, and life sciences.
It's a good sign, though. Humans embracing technology is no longer wishful thinking but reality. ChatGPT has definitely broken the barrier and should be seen as a glimpse of what is yet to come, namely, an exciting future for AI helping humans in meaningful ways.
In Conclusion
You, creators and artists, should not be afraid of ChatGPT! Simply because it has its limitations and because the human touch will always be needed in any piece of content created, from the emotions behind the story of a why to the quotes that certify and differentiate a piece of art. And also because AI ethics will move to the forefront as technology advances and AI researchers become more and more aware of the wrongs that AI can help produce.
I give ChatGPT credits for admitting it.
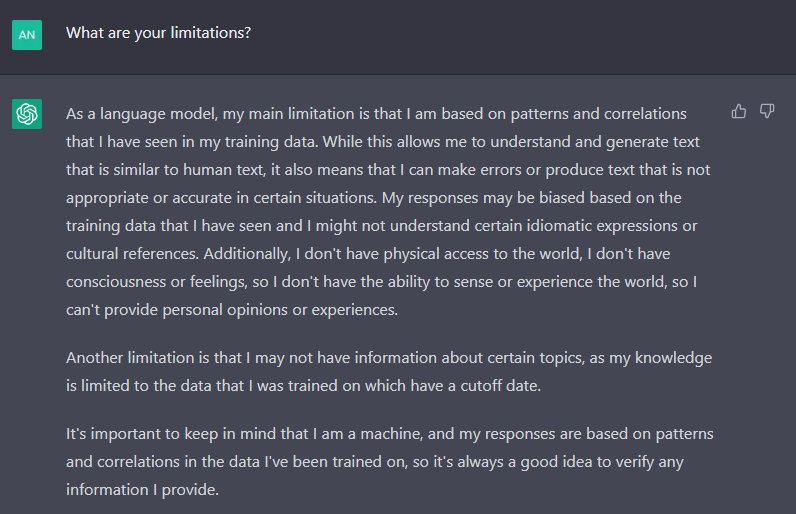
Stay tuned for our next blog post, where we will explore ChatGPT’s "cans" and "cants" and how technologies like conversational AI should leverage LLMs' huge breakthroughs to enhance human potential.